Artificial intelligence (AI) is being used everywhere–from generating human-like conversations to diagnosing diseases. Behind the scenes, the hardware components used for training AI models and facilitating these tasks have distinct benefits and drawbacks. Hardware accelerators for artificial neural networks have emerged as a tool to unlock advanced AI performance, yet conventional computing still struggles with inefficiencies due to memory bottlenecks.
A promising avenue for addressing these limitations is memristors, emerging hardware that functions like artificial synapses and can store and process data efficiently. While memristors hold significant potential for efficient AI acceleration as they enable in-memory computations, their inherent variability also introduces new challenges. A new study led by researchers in GW Engineering’s Department of Electrical and Computer Engineering proposes a novel approach to turning this hardware non-ideality into an advantage: layer ensemble averaging.
“The inspiration for exploring layer ensemble averaging came from observing the inherent variability in memristive devices. Unlike traditional hardware, memristors are prone to stochastic defects, which can significantly impact neural network accuracy,” said Osama Yousuf, a 5th-year Ph.D. candidate in computer engineering and lead study author. “Instead of trying to eliminate these defects entirely–an often impractical approach–our team investigated how to leverage them to improve model robustness.”
Layer ensemble averaging is a hardware-oriented fault tolerance scheme for improving inference performance that enables AI systems to function despite internal errors or disruptions. The study “Layer Ensemble Averaging for Fault Tolerance in Memristive Neural Networks,” published in Nature Communications in February, was conducted in collaboration with researchers from the National Institute of Standards and Technology and industry partner Western Digital Technologies.
While interning at Western Digital last fall, thanks to funding from the NSF INTERN program, Yousuf evaluated large language models (LLMs) under various noise conditions in analog AI accelerators, derived hardware specifications for near-software performance, and performed device characterization on crossbar arrays for ultra-low power inference techniques. These hands-on experiences in industry exposed him to the practical limitations of emerging devices and directly informed the team’s approach.
“Non-idealities and lack of robustness prevents using hardware based on emerging devices, like memristors, for neural network inference because it leads to performance sub-par to software counterparts,” said Gina Adam, an associate professor of electrical and computer engineering, the study’s corresponding author, and Yousuf’s Ph.D. advisor. “Our paper demonstrates a fault-tolerant approach on simple oxide-based memristor devices with a high percentage of defects.”
The research team tested their approach through simulations on an image classification task and hardware experiments using a custom 20,000-device prototyping platform. They found that averaging outputs across multiple redundant layers with different weight perturbations effectively mitigates the impact of individual device failures.
Adam noted that this finding demonstrates promise for ternary weights, making layer ensemble averaging a suitable technique for a wide range of device technologies, such as magnetic tunnel junctions and ferroelectric devices. This versatility strengthens the potential for energy-efficient AI hardware across various domains, from autonomous systems to biomedical devices.
“One surprising discovery was that certain levels of variability improved generalization, similar to regularization effects of dropout in software neural networks. This insight opens new possibilities for designing AI models that embrace, rather than resist, hardware imperfections,” said Yousuf.
Traditional error correction methods often add computational overhead that increases power consumption. By overcoming memory bottlenecks without additional hardware corrections, the team is enabling the design and development of smaller, low-power AI accelerators that could be integrated into edge devices and mobile applications, further expanding the accessibility of emerging AI technologies.
“As the need for AI increases, the field is foreseen to consume exponential computing resources at high environmental and financial costs in the next decade. Therefore, efficient AI operations are critical. Memristors offer promise to provide significantly better efficiency in a compact form factor,” said Adam.
This study is a key part of Adam’s broader research on neuromorphic computing. The prototyping platform was developed in collaboration with NIST and Western Digital with funding from DARPA and ONR. In 2023, she received an NSF CAREER Award, which focuses on entropy-stabilized oxide memristors for software-equivalent neuromorphic computing. One of the main tasks of this project is dedicated to developing transformer architectures based on emerging devices, directly aligning with the NSF INTERN funding that supported Yousuf’s work at Western Digital and enhanced his doctoral research.
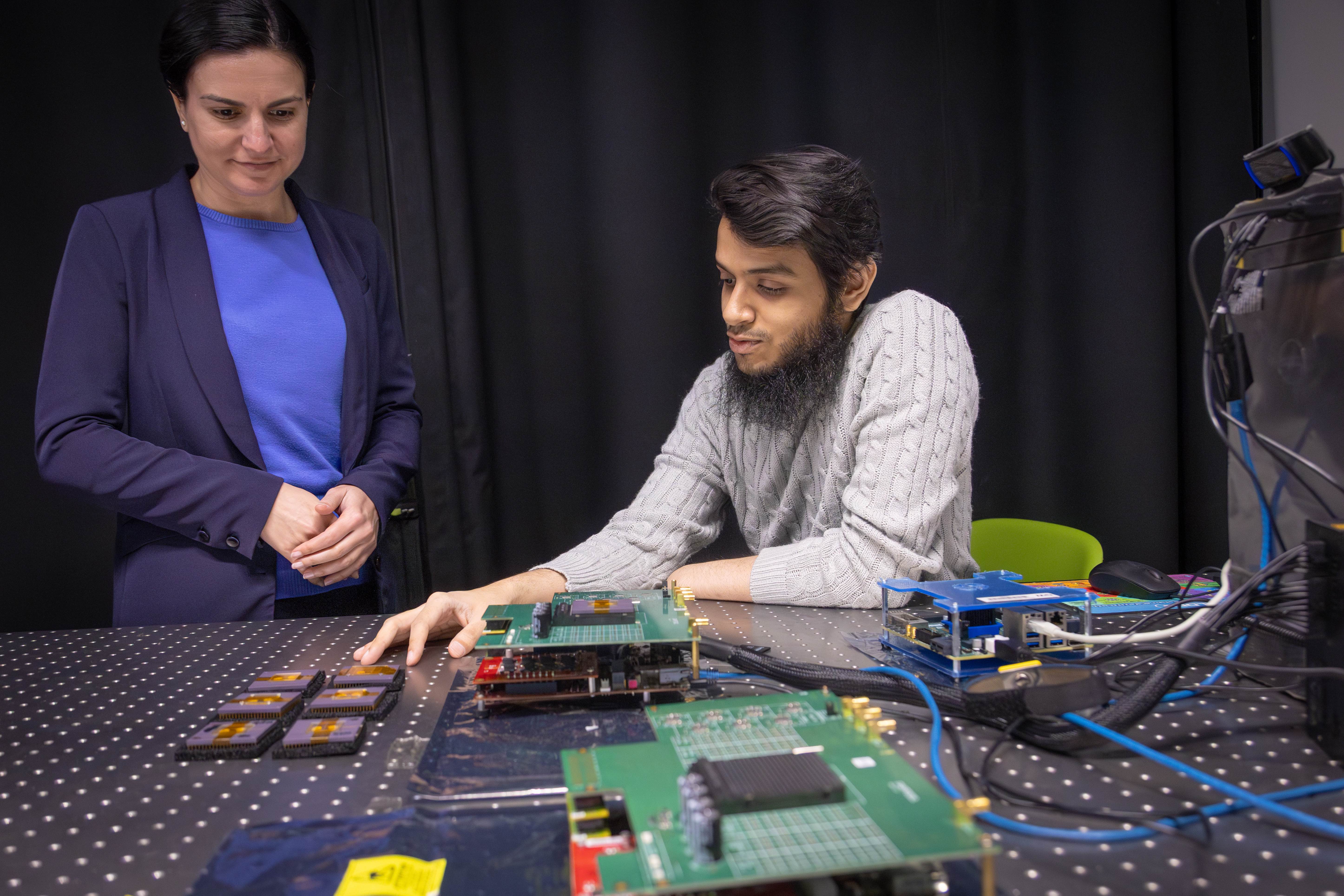
Yousuf’s dissertation focuses on developing robust, energy-efficient neural network algorithms, particularly for in-memory computing architectures, such as memristors. In this study, he played a key role in the entire research process, leading their efforts to develop techniques to improve fault tolerance in neural networks deployed on hardware systems. He also implemented algorithmic improvements to enhance memristive neural network robustness. Now, as he prepares to complete his Ph.D. in May, Yousuf is poised to drive advancements in AI hardware-software co-design throughout his career.
The team’s collaborative efforts push the boundaries of current AI hardware and set the stage for a new era of energy-efficient, resilient, and scalable systems. With even a single AI chatbot response consuming nearly ten times the electricity of a Google search, the innovations led by Yousuf, Adam, and their colleagues are essential for meeting the increasing demand for sustainable computing solutions.